【论文原文】StyleGAN-Wearing
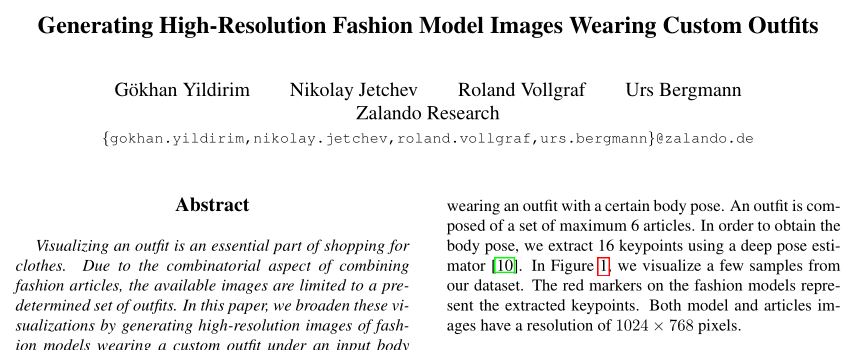
Generating High-Resolution Fashion Model Images Wearing Custom Outfits
Visualizing an outfit is an essential part of shopping for clothes. Due to the combinatorial aspect of combining fashion articles, the available images are limited to a pre- determined set of outfits. In this paper, we broaden these vi- sualizations by generating high-resolution images of fash- ion models wearing a custom outfit under an input body pose. We show that our approach can not only transfer the style and the pose of one generated outfit to another, but also create realistic images of human bodies and garments.
More【论文原文】StyleGAN-Embedder
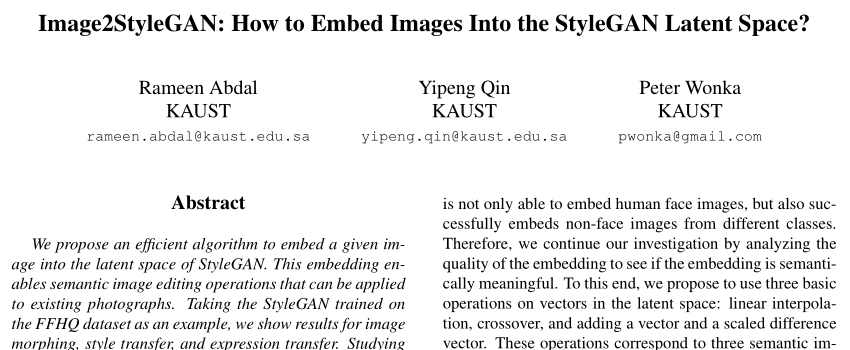
Image2StyleGAN: How to Embed Images Into the StyleGAN Latent Space?
We propose an efficient algorithm to embed a given im- age into the latent space of StyleGAN. This embedding en- ables semantic image editing operations that can be applied to existing photographs. Taking the StyleGAN trained on the FFHQ dataset as an example, we show results for image morphing, style transfer, and expression transfer. Studying the results of the embedding algorithm provides valuable insights into the structure of the StyleGAN latent space. We proposeasetofexperimentstotestwhatclassofimagescan be embedded, how they are embedded, what latent space is suitable for embedding, and if the embedding is semanti- cally meaningful.
More【论文原文】Liquid Warping GAN
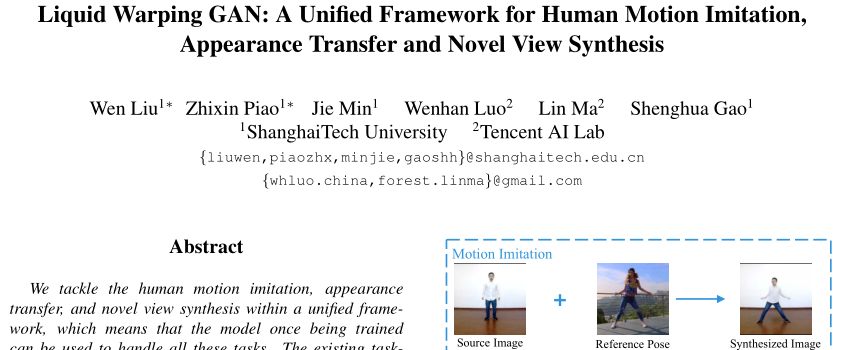
Liquid Warping GAN: A Unified Framework for Human Motion Imitation, Appearance Transfer and Novel View Synthesis
We tackle the human motion imitation, appearance transfer, and novel view synthesis within a unified frame- work, which means that the model once being trained can be used to handle all these tasks. The existing task- specific methods mainly use 2D keypoints (pose) to esti- mate the human body structure. However, they only ex- presses the position information with no abilities to charac- terize the personalized shape of the individual person and model the limbs rotations. In this paper, we propose to use a 3D body mesh recovery module to disentangle the pose and shape, which can not only model the joint lo- cation and rotation but also characterize the personalized body shape. To preserve the source information, such as texture, style, color, and face identity, we propose a Liq- uid Warping GAN with Liquid Warping Block (LWB) that propagates the source information in both image and fea- ture spaces, and synthesizes an image with respect to the reference.
More【论文原文】Few-shot Vid-to-Vid
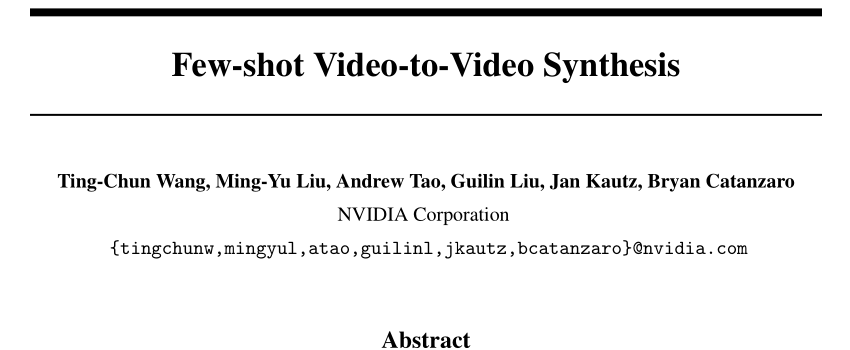
Few-shot Video-to-Video Synthesis
Video-to-video synthesis ( vid2vid ) aims at converting an input semantic video, such as videos of human poses or segmentation masks, to an output photorealistic video. While the state-of-the-art of vid2vid has advanced significantly, existing approaches share two major limitations. First, they are data-hungry. Numerous images of a target human subject or a scene are required for training. Second, a learned model has limited generalization capability. A pose-to-human vid2vid model can only synthesize poses of the single person in the training set. It does not generalize to other humans that are not in the training set. To address the limitations, we propose a few-shot vid2vid framework, which learns to synthesize videos of previously unseen subjects or scenes by leveraging few example images of the target at test time.
More【论文原文】StyleGAN2
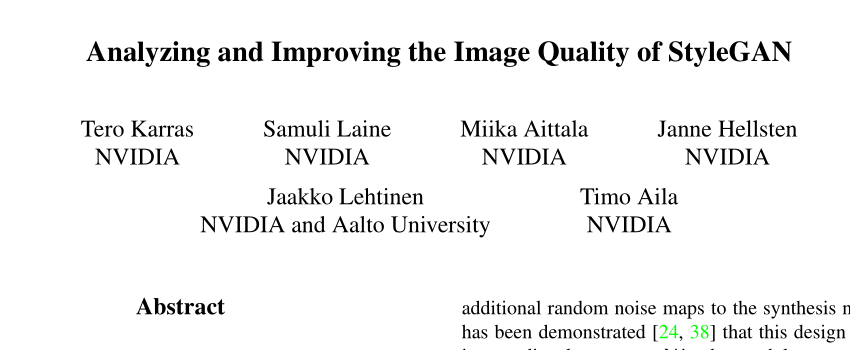
Analyzing and Improving the Image Quality of StyleGAN
The style-based GAN architecture (StyleGAN) yields state-of-the-art results in data-driven unconditional gener- ative image modeling. We expose and analyze several of its characteristic artifacts, and propose changes in both model architecture and training methods to address them. In par- ticular, we redesign generator normalization, revisit pro- gressive growing, and regularize the generator to encour- age good conditioning in the mapping from latent vectors to images. In addition to improving image quality, this path length regularizer yields the additional benefit that the gen- erator becomes significantly easier to invert. This makes it possible to reliably detect if an image is generated by a par- ticular network. We furthermore visualize how well the gen- erator utilizes its output resolution, and identify a capacity problem, motivating us to train larger models for additional quality improvements. Overall, our improved model rede- fines the state of the art in unconditional image modeling, both in terms of existing distribution quality metrics as well as perceived image quality.
More【论文原文】SinGAN
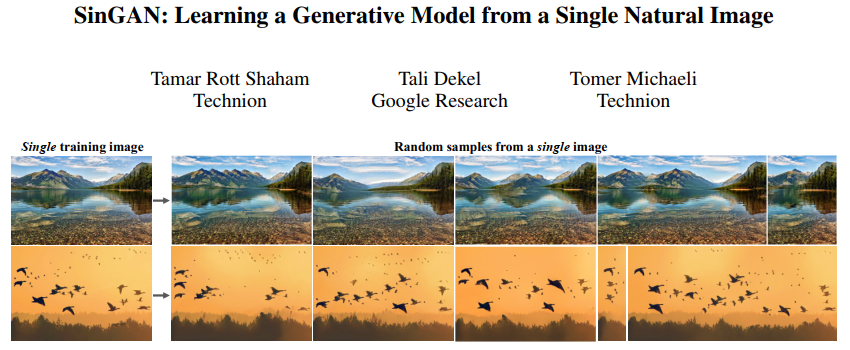
SinGAN: Learning a Generative Model from a Single Natural Image
We introduce SinGAN, an unconditional generative model that can be learned from a single natural image. Our model is trained to capture the internal distribution of patches within the image, and is then able to generate high quality, diverse samples that carry the same visual content as the image. SinGAN contains a pyramid of fully convolutional GANs, each responsible for learning the patch distribution at a different scale of the image. This allows generating new samples of arbitrary size and aspect ratio, that have significant variability, yet maintain both the global structure and the fine textures of the training image. In contrast to previous single image GAN schemes, our approach is not limited to texture images, and is not conditional (i.e. it generates samples from noise). User studies confirm that the generated samples are commonly confused to be real images. We illustrate the utility of SinGAN in a wide range of image manipulation tasks.
More【论文原文】U-GAT-IT
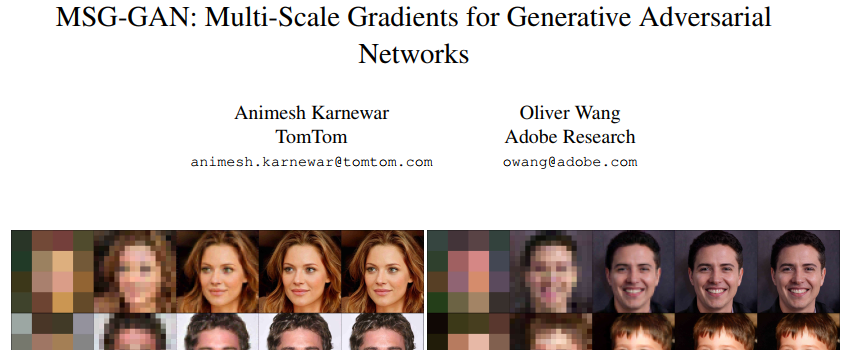
U-GAT-IT: Unsupervised Generative Attentional Networks with Adaptive Layer-Instance Normalization for Image-to-Image Translation
We propose a novel method for unsupervised image-to-image translation, which incorporates a new attention module and a new learnable normalization function in an end-to-end manner. The attention module guides our model to focus on more important regions distinguishing between source and target domains based on the attention map obtained by the auxiliary classifier. Unlike previous attention-based method which cannot handle the geometric changes between domains, our model can translate both images requiring holistic changes and images requiring large shape changes. Moreover, our new AdaLIN (Adaptive Layer-Instance Normalization) function helps our attention-guided model to flexibly control the amount of change in shape and texture by learned parameters depending on datasets.
More【论文原文】MSGGAN
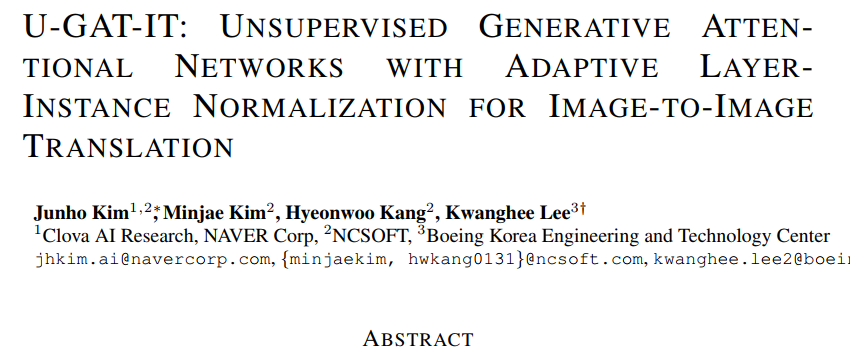
MSG-GAN: Multi-Scale Gradients for Generative Adversarial Networks
While Generative Adversarial Networks (GANs) have seen huge successes in image synthesis tasks, they are notoriously difficult to adapt to different datasets, in part due to instability during training and sensitivity to hyperparameters. One commonly accepted reason for this instability is that gradients passing from the discriminator to the generator become uninformative when there isn’t enough overlap in the supports of the real and fake distributions. In this work, we propose the Multi-Scale Gradient Generative Adversarial Network (MSG-GAN), a simple but effective technique for addressing this by allowing the flow of gradients from the discriminator to the generator at multiple scales.
More【论文原文】SMIS
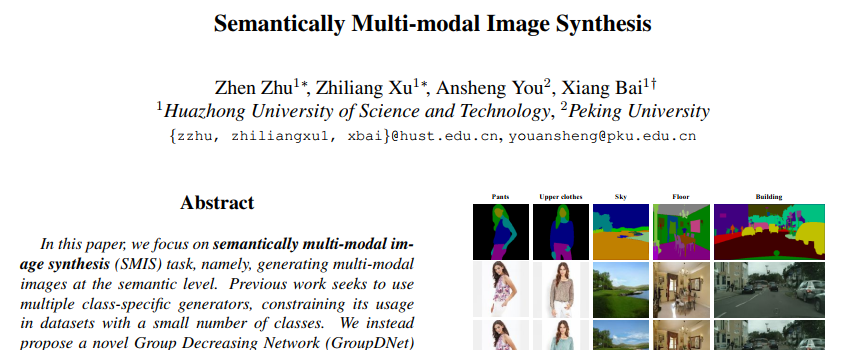
Semantically Multi-modal Image Synthesis
In this paper, we focus on semantically multi-modal image synthesis (SMIS) task, namely, generating multi-modal images at the semantic level. Previous work seeks to use multiple class-specific generators, constraining its usage in datasets with a small number of classes. We instead propose a novel Group Decreasing Network (GroupDNet) that leverages group convolutions in the generator and progressively decreases the group numbers of the convolutions in the decoder. Consequently, GroupDNet is armed with much more controllability on translating semantic labels to natural images and has plausible high-quality yields for datasets with many classes.
More